Industry Insights
4 Use Cases For AI
Here's four essential AI use cases that business leaders should consider today.
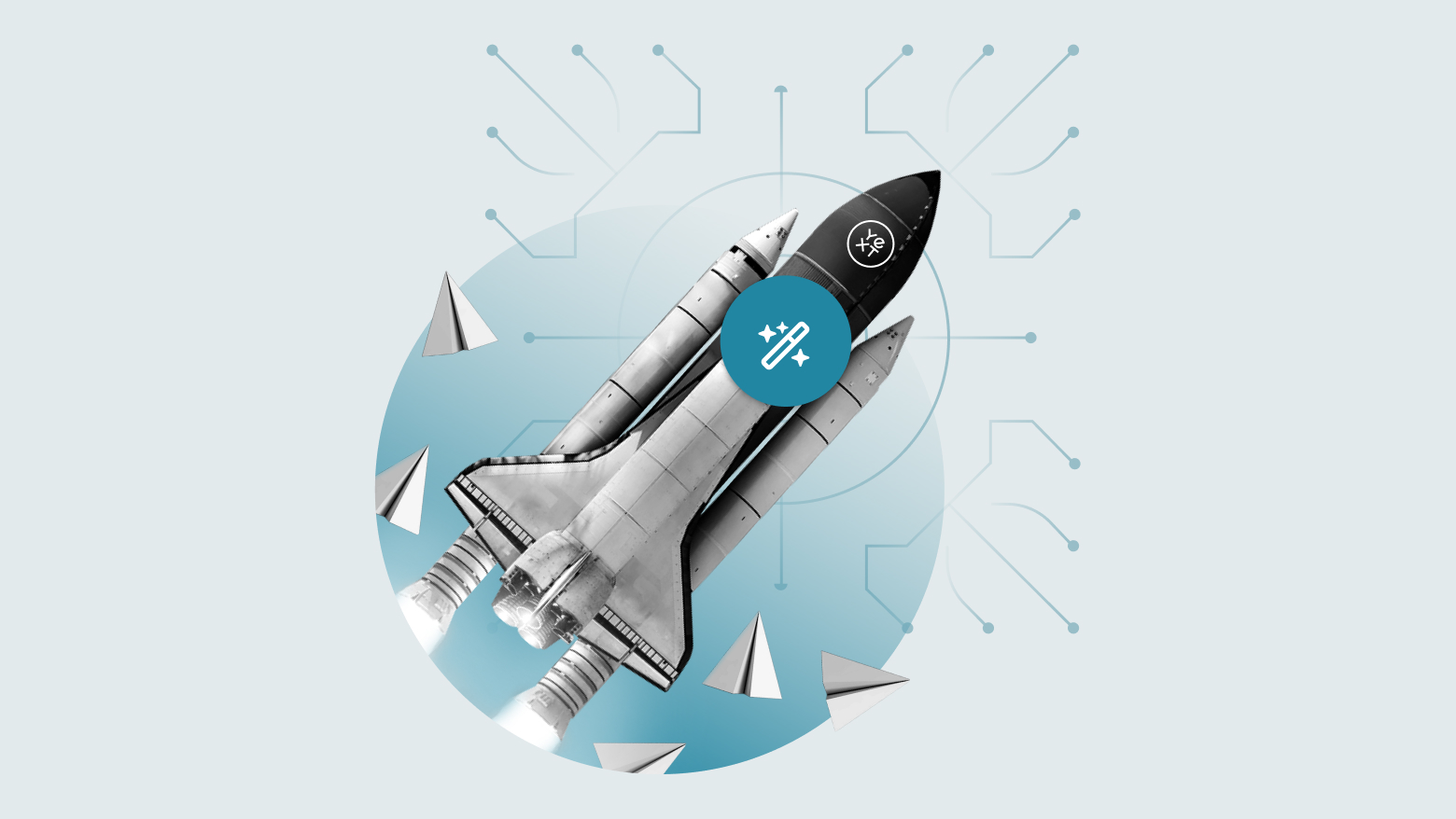
The rise of generative AI— and especially large language models like GPT-3 and ChatGPT — has been the most important advancement in AI in recent years. Today, these models are much larger and more intelligent than their predecessors, and they can perform tasks simply by being prompted rather than requiring extensive training datasets. This makes them a game changer for businesses and consumers alike, allowing for new types of automation and digital experiences that were previously impossible. In other words, it makes possible things that would have been considered science fiction only a few years ago. And, used properly, it has the potential both to create enormous value for the economy and genuinely improve peoples' lives. But to fully realize the potential of AI, it's essential to understand its strengths and its limitations, and to use it responsibly and effectively. Here's a look at the four essential AI use cases that business leaders should be learning about today. Prefer to read the full guide? Click here.
- AI as creative assistant One of the most promising use cases for AI is as a creative assistant, where it helps high-level creative employees with suggestions, information retrieval, and output support. This is already happening with products like GitHub Copilot and ChatGPT, and we can expect more products to emerge in different industries like law, medicine, advertising, entertainment, finance, and academia. Importantly, the human is still in control and gets to accept, reject, or tweak the AI's suggestions.
- AI as business process outsourcing Many business processes will be candidates for automation in the coming years, such as classifying and routing support tickets, medical coding, and AML/KYC processes. LLMs (large language models) like GPT-3 raise the bar for what can be automated while lowering the technical barrier. However, outsourcing a process completely to AI is challenging because there is no longer a human directly in the loop. The requirements for accuracy become much higher, and additional safeguards are needed to monitor and retrain the model over time if this option is pursued.
- AI as digital experience A third use case is using AI to create or augment digital experiences. This use case is already well-established. Most people have already been interacting with AI-powered digital experiences for years now. We use an AI-powered search engine every time we interact with Google. We use AI-powered recommendation engines to browse movies, songs, and products on Netflix, Spotify, and Amazon. We use AI-powered conversational agents like Siri or Alexa to look up information and perform tasks. AI is increasingly the medium through which we interact with digital businesses. When it comes to AI-powered digital experiences, we usually aren't trying to replace a human but rather provide an experience that can only be delivered by software and AI. The human's role — to the extent that there is one — is to monitor the AI's behavior and curate the content that it has access to.
- AI as prediction and forecasting The final use case is one that you won't read about as often in the press, but it is extremely valuable: AI can be used to predict the future. Astute readers will point out that all AI is predictive: LLMs are predicting the next word in a sequence, diffusion models are predicting the ideal set of pixels for an image, recommendation engines are predicting whether a user will like a product. In this case I'm referring specifically to predicting business outcomes in the real world. For example:
- Predicting consumer demand for a specific product based on historical trends
- Predicting what the stock market will do tomorrow based on a variety of factors
- Predicting the weather tomorrow based on IOT data You might think of these things more so as "statistics" than "machine learning" or "artificial intelligence," but, as it turns out, those are all the same thing. AI and machine learning are statistics, applied at an extraordinary scale and to problems that we don't traditionally think of as involving numbers. Of course, this is just the tip of the iceberg when it comes to AI, and we're likely to see vast growth in these use cases — and more — in the coming months and years. Further, as with any new technology, there are risks and challenges that need to be addressed: bias, privacy, and job displacement among them. But if used responsibly and effectively, AI has the potential to create enormous value for businesses and individuals alike, improving our lives in ways we can't even imagine yet. Ready to dive deeper? Click here to download the full Four Essential AI Use Cases guide.**