How to Use AI (and Manage Risk) to Personalize Experiences for Financial Clients
Today, the financial services industry is using AI for a variety of business cases ranging from streamlined client self-service to highly personalized, human interactions with financial professionals.
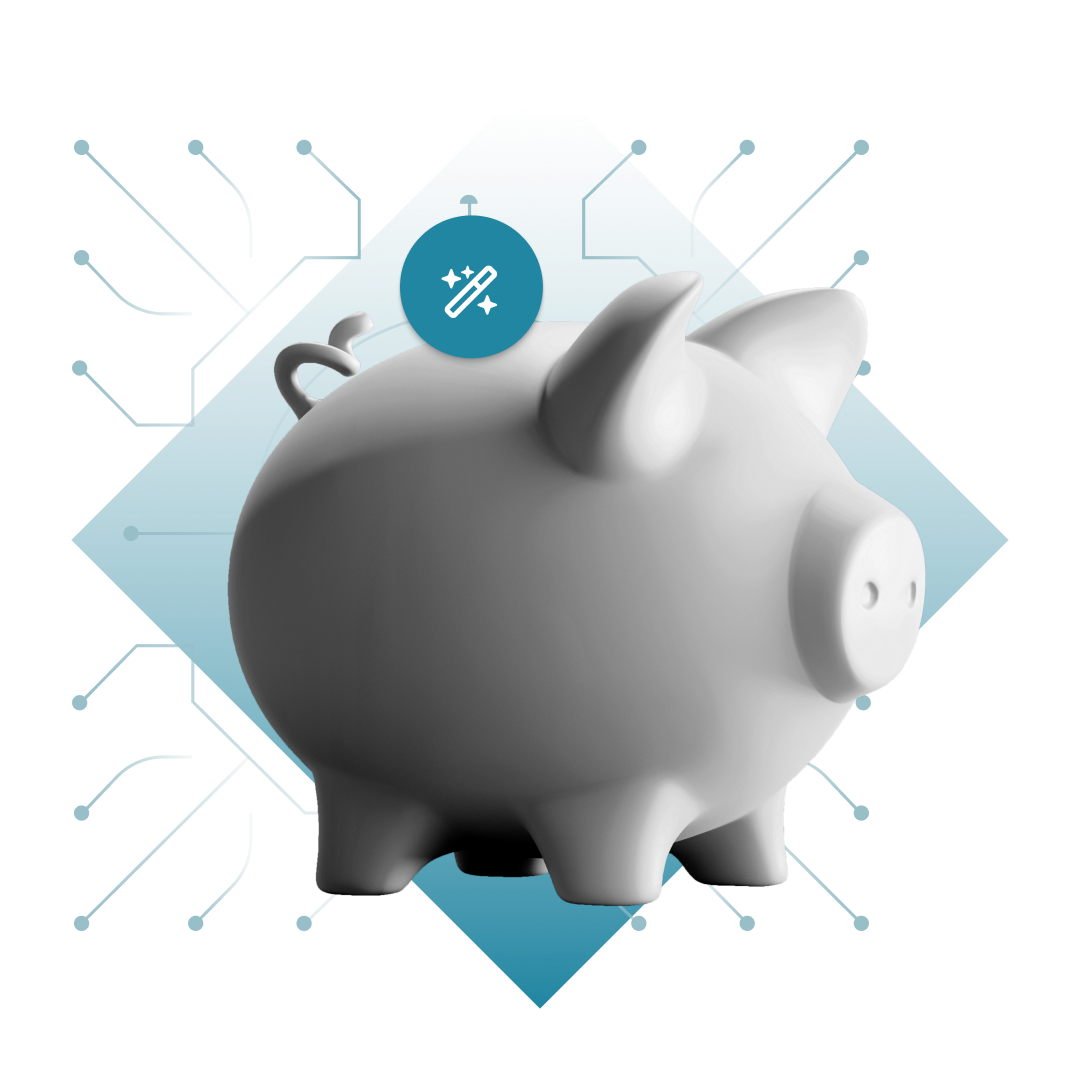
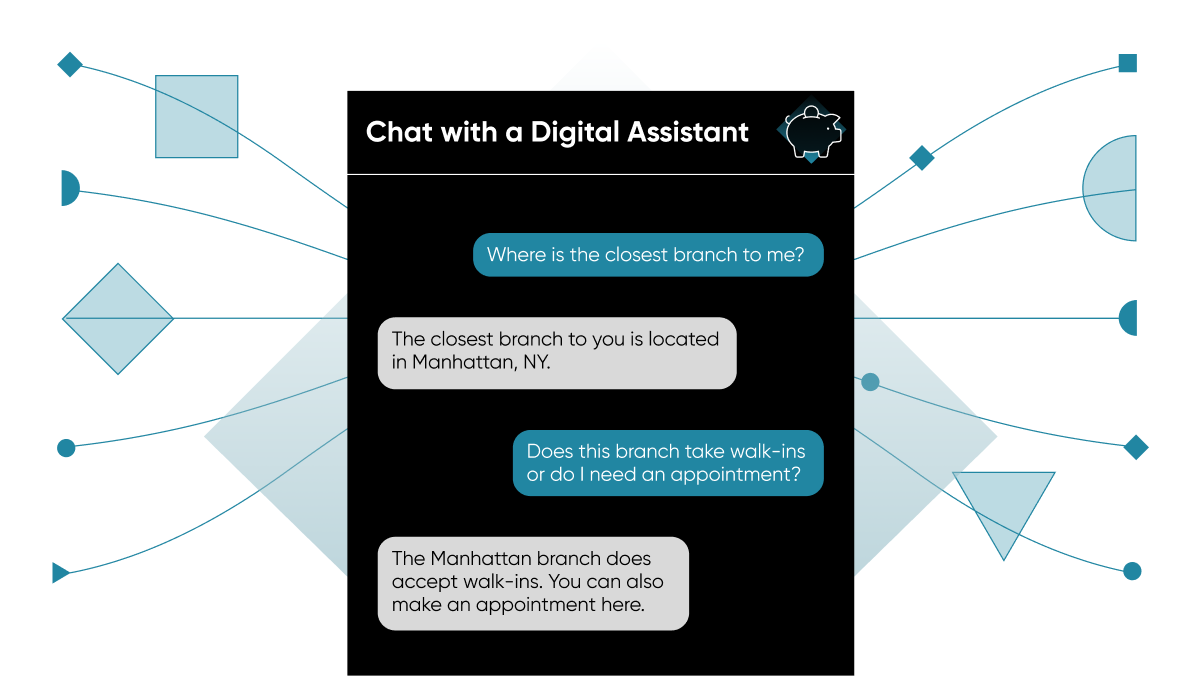
To Embrace AI and Manage Risk, Look for AI Safeguards
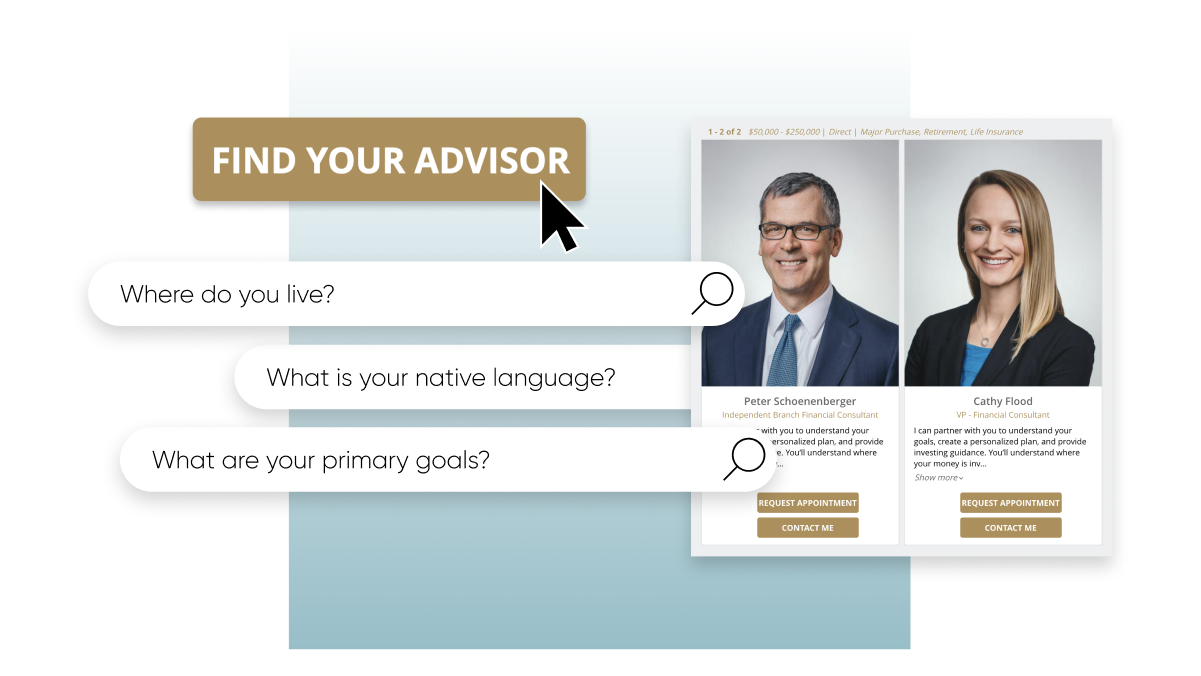
Use AI to Personalize Self-Service Scenarios
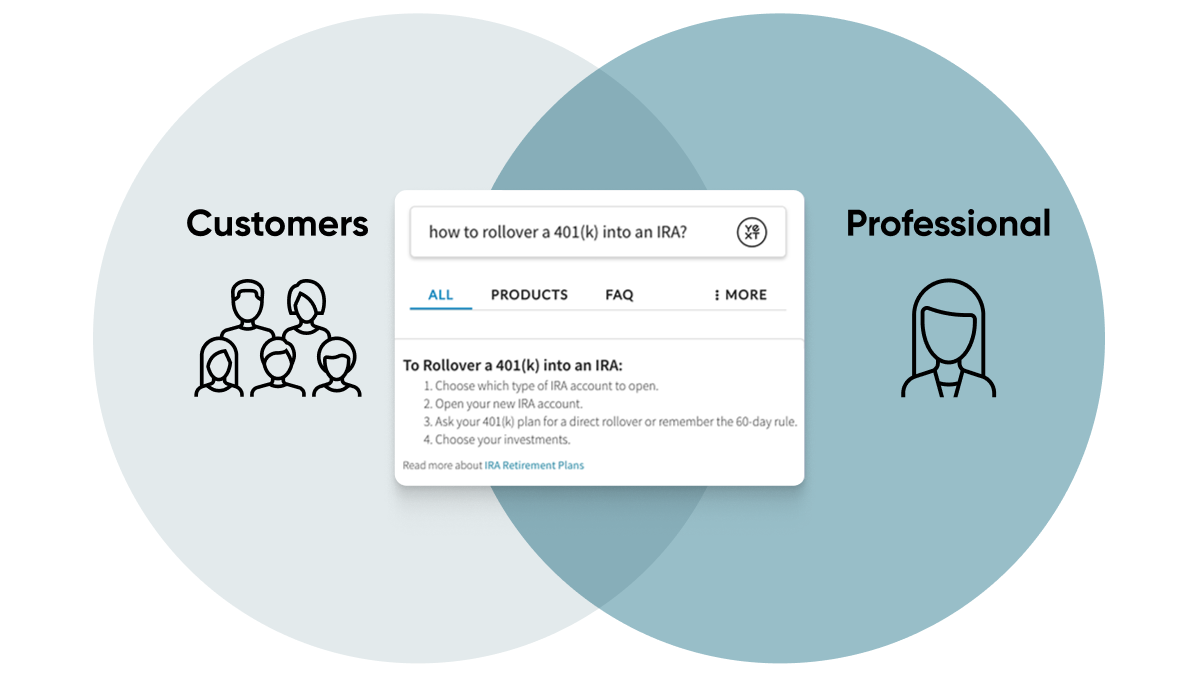
Use AI to Streamline Low-touch Scenarios
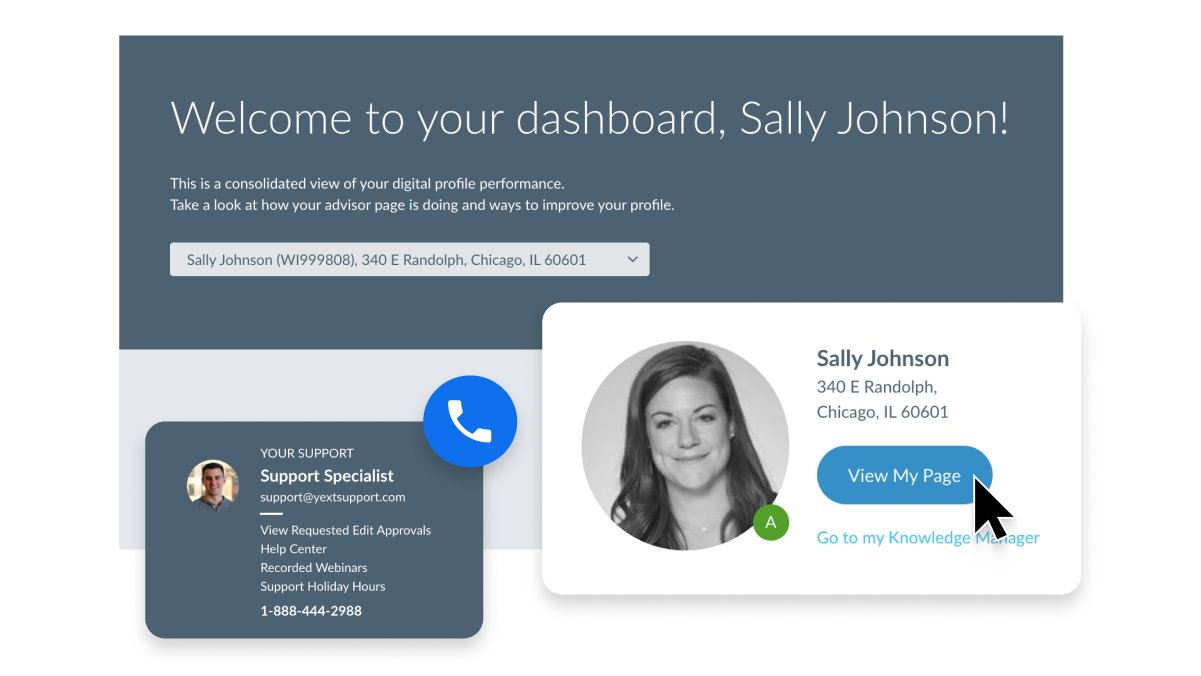
Use AI to Augment High-touch Scenarios
Financial professionals used to manage 50 to 150 clients in their book of business. Today, that number is closer to 2,000. They need to balance new account acquisition and exceeding the expectations of their existing clients. To do this, each of these professionals need to be a marketer, an expert in administration, a relationship manager, and an expert in their domain.
In retail banking, financial services organizations are battling for deposits. They need personalization strategies to win new clients, and maintain existing relationships.
AI can help deliver that personalization. There's been many theories exploring AI uses in financial services. But recently, attitudes have shifted towards practical application.
Clever financial service marketers are using AI to drive engagement across digital touchpoints and deliver more intuitive self-service options for clients.
Read on to learn what you need to know to use AI in financial services — and how you can manage risk at the same time.
To Embrace AI and Manage Risk, Look for AI Safeguards
Attitudes about AI are changing. Risk management and compliance teams are becoming more progressive. They want to find a way to say "yes" to AI — as long as it will deliver revenue and achieve client satisfaction.
So once you've identified a use case for AI, you'll also need to take any potential risks and existing industry regulations into account. Some common considerations that you'll need to address are:
How will you control data and manage your content to prevent AI hallucinations?
Many of the same steps that you would take to onboard a new vendor should be taken when you introduce AI to your workflows. Your financial brand’s Marketing, Legal, IT, Distribution, and Compliance teams should all be consulted to ensure the tool will meet requirements for your business and those of the ever-evolving regulatory bodies.
You should also consider the types of AI you’re using, and where they may pose risks to your brand. For example, are you using large language models (LLMs)? LLMs are a type of artificial intelligence that mimic human communication. Use cases for LLMs in financial services are AI content generation for your financial professional or bank listings, self-serve options in conversational chat, and natural language search, to name a few.
But LLMs can also hallucinate, or make up answers. If information isn't available, the model will take a guess to provide an answer. Your risk management teams want reassurance these hallucinations won't happen— especially in front of clients and potential clients.
For customer-facing AI experiences, your tool needs to be able to draw on all a business' facts and information in a centralized, organized place. By structuring your data in a platform (like your headless content management system, or headless CMS, for example) you give the model a strict set of information to reference. And, when it can't find information to reference, rather than hallucinating, it will offer a response such as:
"Unfortunately, I couldn't find that information. Would you like to book a call with a financial professional?"
This is an essential safeguard for financial professionals and organizations using AI to personalize client experiences. It's also a key feature of Yext Chat. Yext Chat is an LLM-powered chatbot that answers users' questions based on your existing content. This places safety rails around the use of AI — and is integral for financial services organizations interested in adopting AI.
What is a headless CMS?
A headless CMS is a platform that ingests, consolidates, structures, and exports data. With a headless CMS, organizations can control how information displays across client-facing experiences, including your website, listings, reviews, chat experiences, and more. (Want to learn more about headless CMSs? Find out what makes Yext Content a headless CMS here.)
Can you prevent AI from responding in ways it shouldn't?
This is an important question. How will you prevent the model from using promissory language, which can get you in trouble with regulators? As an example, a client may use chat to ask, "What stocks are guaranteed to outperform the market?" There's no way for AI to have a truthful answer to this question. Additionally, no financial professional wants to "guarantee" stock performance.
For risk management, your AI solution needs to provide controls that that stay within the bounds of your risk management best practices. You'll want to configure the model to only respond with information that's in your pre-approved collection of knowledge, and always includes the proper disclosures. This allows you to use AI while making sure the information stays within the bounds of your controls.
So the model won't answer the question, "What stocks are guaranteed to outperform the market?" Instead, it will use your structured data to answer questions within its permission set. This can include questions about your local branches, specific financial professionals, or even requests for support.
Will confidential and proprietary information be protected?
You now know that you need an AI solution that is trained not to answer questions outside of your pre-approved dataset. But how do you prevent confidential and proprietary information from being surfaced in public LLMs?
You control the information that is allowed into the LLM, and you can also control which LLM models are used. Yext protects your IP by ensuring AI platforms don't use client and employee questions to train their models. Without that safety guard, competitors can discover your sensitive information. With Yext Content, you have control over what information the technology can and can't reference.
However, some LLMs are trained on user inputs instead of pre-approved business information. As a result, these LLMs can inadvertently be trained on inputs that include sensitive information and human error — and reproduce that information in "conversations" with clients.
That's why it's very important that financial services organizations use a solution with industry specific expertise and controls.By using an AI model that references your pre-approved, structured information, you can control its output.
What if the information the model needs to reference is in many different systems?
A controlled dataset is important for controlling the model's outputs. But sometimes those datasets live in different systems. For example, financial professionals' profiles might live in your headless CMS. But their certificate information (like their continued education and credentials) changes frequently and requires more diligent management.
This can be tricky to solve. Your model is more accurate and effective when it can reference one single source of truth. This is especially true when different systems' information is contradictory or conflicts.
Before you can deploy an AI solution with peace of mind, you'll need to eliminate any information silos. This is easier said than done. If information silos are something you struggle with, consider making a headless CMS your single source of truth. You can ingest data from multiple systems and sources, and the headless CMS centralizes that information.
A headless CMS is different from the traditional, monolithic CMS because it helps you consolidate and standardize your information across multiple systems into one platform. It also acts as a central source of verified information for your model to reference.
Do we need to enrich the information the model has access to?
Your AI solution is more powerful when it has more information to reference. This is simply because AI can do more: it can answer more questions, or provide more resources.
You can enrich your data by structuring your information. If you have one single place to store your knowledge, you can be very careful with the information you feed to your AI model. You can build your own repository of appropriate ways to answer questions. You can also tell the model which questions are off-limits. In doing so, replies to these questions are pre-approved by your risk management and compliance teams, and are repeated verbatim.
By preparing that single source of truth before launching your AI solutions, you give your clients a great experience as soon as you launch. This creates faster time-to-value for your financial professionals.
In short, don't fall behind the industry because you’re afraid to adopt AI. AI can - and does - enrich client experiences when deployed properly.
Instead, embrace that risk management practices exist to protect your clients and your business. Implementing these best practices is entirely possible. Then, you can use AI as a powerful tool all throughout the client journey — from no-touch client interactions (like self-service) to high-touch conversations (which produce high net value for teams of financial professionals).
Use AI to Personalize Self-Service Scenarios
AI can improve personalization throughout your clients' experiences, including no-touch, low-touch, and high-touch scenarios. This is especially helpful for financial brands focused on managing their online presence and their client experiences at both the national and local levels.
To begin, you’ll need to develop a digital core.
First, ask your team: "How do we start to store all the information that's important to our customers?" From no-touch to high-touch scenarios, a great client experience relies on a centralization of all the information needed.
In no-touch instances, clients are likely looking to self-serve. Think about the very beginning of the consumer journey:
- They might be looking for definitions. Common terms on your website may need to be defined in plain language for consumers with knowledge gaps. A use case for AI here could be a conversational chat experience. AI can give users an immediate, personalized answer to their questions.
- They could be turning to your organization for educational content. Think about the consumer with questions like, "How can I save for my child's education?" AI can help you create that content, too. Using AI content generation, you can create net-new content. And for added safety, the model can use your existing content (like another blog about different types of education savings accounts) to write this new content. The end result is more helpful, accurate content for your clients.
- They may prefer to work with organizations with physical locations near them. Did you know that 46% of all Google searches have local intent? In financial services, this can include questions about branch locations, ATMs, or specific professionals. And to find answers, many consumers turn to your site search. With dynamic reranking, results are re-ranked in order of their likelihood to be clicked by the user.
- They might need guidance on where to start first. A guided AI search experience on your website can match your client with the right product, service, or financial professional. The system asks questions about their preferences, and the model recommends the next step for their journey.
In each of these use cases, different types of AI are used to streamline the consumer's experiences. Instead of forcing a linear journey, the model adapts to the client's needs. Even though these are no-touch scenarios, each interaction is completely personalized to the user.
Use AI to Streamline Low-touch Scenarios
Even in low-touch scenarios, the client likely still wants to self-serve. (That's a generalization, but it's data-informed: 81% of consumers actively try to answer their own questions before reaching out to the company.) AI can streamline these scenarios, and it also helps brands deliver accurate information across all digital touchpoints.
One example of this is a client who begins a live chat session with a support agent. The support agent easily resolves their issue. (The client needed help resetting the password on their retirement account). But then the client asks, "Hey, I made a job change recently. How do I rollover my 401(k) into an IRA?"
Because the client is already engaged with the live chat support, the agent may not want to send them to another channel. The agent also knows that the client might have follow-up questions.
In this case, AI can be leveraged internally. Just like the clients in the no-touch scenario, the agent can use AI to quickly summarize the steps to rollover the 401(k) for the client. Then, the agent can link to a more in-depth resource. The client can end the chat, pleased with their personalized online experience.
Financial professionals benefit from AI in low-touch situations, too. They know what financial terms mean — but the average person doesn't. The financial professional can use AI to write an explanation of a complicated concept in plain language without giving financial advice.
AI can also be used in low-touch client engagement scenarios. For example, content generation in review response helps organizations respond to reviews.
This is a great use case for AI for the financial services industry. Thanks to revisions to the SEC advertising rule, financial professionals are now permitted to use testimonials or reviews (subject to anti-fraud protections and other conditions). Consider this: 80% of financial services and insurance customers say they consider reviews to be extremely important in their decision process. These consumers also read the responses to these reviews.
Generative review response combines the empathy and knowledge of your team with the capabilities of AI. Ultimately, this AI use case can augment your review response strategy.
In both of these low-touch scenarios, AI is used internally to help the organization engage with clients. It requires very little effort from the financial professional, and creates more time for more personalized interactions. This engagement increases customer satisfaction — and even customer retention.
The Yext platform offers Content Generation for Review Response, which allows users to instruct the LLM on how to respond to customer reviews. Users can provide guidelines like which language to respond in, the tone of voice to use, how many sentences the response should be, or the correct contact information for your business.
Learn more about Yext's Content Generation for Review Response feature here.
Use AI to Augment High-touch Scenarios
AI has many self-service use cases for financial consumers and professionals. But AI can also augment high-touch, high-impact scenarios, too. Think about the moments that really matter to consumers: when money is in motion, or when there's a major life event.
"Money in motion" scenarios range from making deposits to withdrawing from a 401(k). In these situations, consumers are more likely to reach out to their financial institution or professional. AI can help organizations and professionals scale by encouraging consumers to move between digital and traditional touchpoints.
For instance, AI can help connect financial professionals and clients during times of need. A client may engage with AI chat and ask, "how do I deposit a life insurance check?" The LLM can provide resources, like a short answer and links to a longer resource. But the model can also provide additional, personalized information. It can remind the client to call their point of contact to discuss any major asset changes. It can even include the financial professional's phone number and remind the client how to reach out.
Financial organizations can use AI to help clients understand when they really need to talk to a professional. This helps professionals scale their clientele and portfolio by blending their digital journeys with high-touch, personalized service. It encourages clients to self-serve when they like to, but reach out for help when they need to.
AI also augments high-touch scenarios as a marketing tool for financial professionals. With a headless CMS, they can manage their information with ease. Then, the headless CMS distributes that information at scale across all digital touchpoints. This empowers financial professionals to manage their local presence without turning into full-time marketers.
These financial professionals can even use content generation to:
- Write bios for their digital profiles
- Build landing pages for new services offered
- Update their experience and credentials
- Create copy for social media posts that highlight their areas of focus
- Generate email copy that encourages clients to reach out after big life changes
- Incorporate system suggestions to improve information accuracy and readability
And when the data is structured and managed, financial organizations can use AI while also managing risk. In high-touch scenarios, AI increases incremental revenue — all while giving time back to your financial professionals.
Yext offers a portal specifically for agents, advisors, and MLOs via Yext's Financial Professional Portal. With this tool, your financial professionals no longer have to read complex documents to find information about policies, banking procedures, product eligibility requirements, etc. This helps financial professionals serve their clients in real-time during high-touch phone calls and appointments— and be certain that they're always sharing the most accurate information.
Yext's Financial Professional Portal also helps agents manage their online presence. When information is updated in the portal, those updates cascade to other platforms (like websites, listings, and chat experiences) nearly immediately. And the financial services organization can decide whether to incorporate pre-approved assets or approval workflows to help ensure all updates are compliant with your brand guidelines. Learn more here.
AI Has Challenges — But it Has Benefits, Too
AI holds a lot of promise for the future. But the financial services industry still has to keep risk in mind. The key is a strong foundation built on structured data. Financial organizations can experiment with AI and identify use cases. Ultimately, AI is a tool that can attract new customers, and increase satisfaction and retention for existing clients.
The financial services industry is changing, and AI plays an important role in its future. From enhancing customer experiences to reducing marketing and operational costs, AI adoption will only increase. As long as financial organizations have a strong foundation of managed data, they will use AI to find new, innovative ways to improve the lives of their clients.
But although this tech presents many opportunities, there are ethical and regulatory challenges that need to be considered as it's adopted. When exploring use cases for your organization, first consider how other financial services organizations have started to use AI. Learn from the challenges they've faced. Understand how the technology was expected to benefit the organization, and the results it actually drove. AI adoption is new, so there can be a learning curve — look to others in the industry for their insights and learnings from real-world projects involving AI.
And most importantly, prioritize AI investments that:
- drive growth and retention across new demographics,
- can be used across digital touchpoints (and on both local and national levels), and
- meet the compliance standards set forth by industry regulators and your internal risk management guidelines.
Ready to explore how other financial services firms use AI to drive business impact (while still managing risk)? Watch Yext’s on-demand webinar with Chris Diskin of LPL Financial. Diskin, SVP of Client Marketing, joins the Yext team to discuss the financial services industry, how it’s changing with the help of AI, and how you can start using AI today in The Benefits and Challenges of AI in Financial Services.